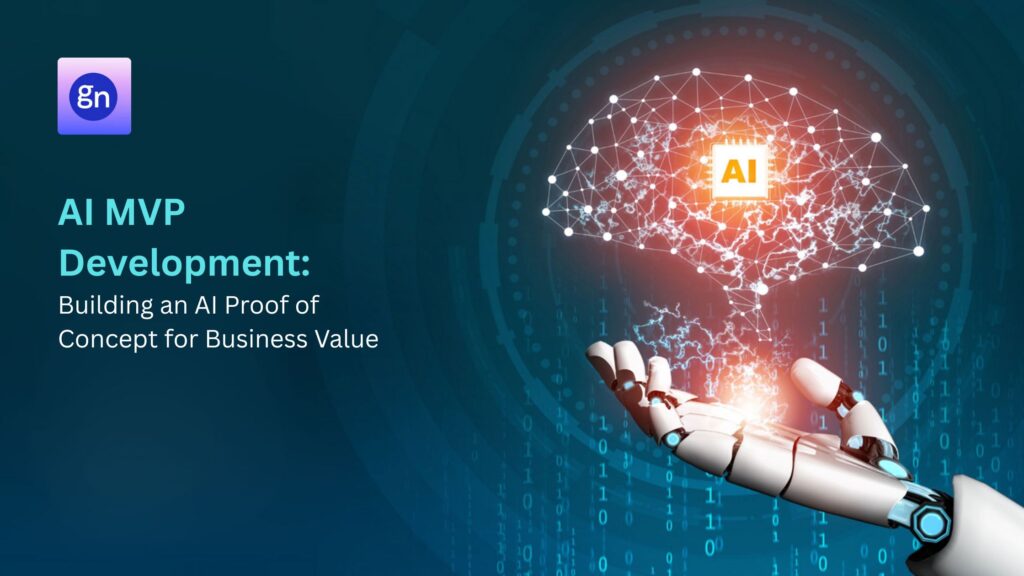
The journey from an AI idea to a production-grade system is long, complex, and full of uncertainty. That’s why smart organizations start by building an AI MVP — an AI Minimum Viable Product. An AI MVP is a functional proof of concept that focuses only on the core AI capabilities necessary to validate technical feasibility and business value.
In this guide, we will explain what an AI MVP is, why it matters, how to build one, and the steps you need to follow to deliver a strong AI proof of concept.
What Is an AI MVP?
An AI MVP is a stripped-down version of an AI-powered application that contains only the essential machine learning or intelligent features. It allows teams to test the solution with real users, real data, and real-world scenarios without investing the time and cost required for a full-scale deployment.
Unlike a traditional software MVP that focuses on feature validation, an AI MVP focuses on:
- Proving if the AI models work on real data
- Demonstrating the user experience around AI decisions
- Validating the business case through early results
In short, AI MVP development bridges the gap between experimentation and commercial success.
Why Building an AI MVP First Is Critical
- Validate Technical Feasibility
Not every AI idea is technically viable. An AI MVP helps assess if models can achieve the accuracy, performance, and reliability needed for production. - Prove Business Value Early
Before committing full resources, you need evidence that your AI solution can solve a real problem and generate returns. An AI MVP provides this validation. - Reduce Time and Budget Risks
Instead of investing six to twelve months in a full-scale product, you validate assumptions quickly with a lean, focused prototype. - Gather Real User Feedback
User interactions with an AI MVP reveal usability issues, gaps in logic, and unexpected edge cases early in the process. - Attract Internal or External Buy-In
Showing a working AI prototype makes it easier to secure stakeholder support or additional funding for scaling.
Key Steps in Building a Successful AI MVP
1. Define a Clear Problem Statement
AI should solve a real problem, not just add technology for technology’s sake. Before any development begins, define:
- What problem are you solving?
- Who are the users?
- What decision or prediction is the AI expected to make?
Be specific. Vague problem statements lead to vague MVPs.
2. Identify Core AI Features
An AI MVP must focus only on the essential intelligent functionality. Cut out every non-essential feature or model enhancement that can wait.
Ask:
- What is the minimum AI functionality needed to prove the concept?
- Can basic rules, heuristics, or lightweight models simulate the experience at first?
Remember, simpler models can still be powerful at the MVP stage.
3. Select the Right Data
Good AI depends on good data. For the AI MVP stage:
- Use real-world datasets whenever possible
- Prioritize quality over quantity
- Understand what data preprocessing is required
- Simulate data if necessary, but validate assumptions early
If the data is noisy, sparse, or biased, the AI MVP should still reveal those limitations upfront.
4. Build a Rapid Prototyping Stack
Speed matters in AI MVP development. You don’t need a production-grade, highly scalable system for your proof of concept.
Typical tech stack for an AI MVP:
- Cursor for rapid coding and model testing
- Lovable for fast and cost-effective data labeling
- Python, TensorFlow, or PyTorch for core model development
- Streamlit, Flask, or simple React front-ends for interface
- Cloud platforms like AWS or GCP for scalable training and deployment
Keep infrastructure simple, portable, and easy to tear down or rebuild.
5. Focus on Evaluation Metrics
Success criteria for your AI MVP must be measurable. Examples include:
- Model precision and recall thresholds
- Response time for predictions
- User satisfaction scores with AI interactions
- Operational KPIs impacted (e.g., hours saved, errors reduced)
Define your evaluation metrics before coding. This way, you know exactly when the MVP is a success—or needs rework.
6. Conduct User Testing Early
Deploy your AI MVP in a controlled environment with a small user group. Observe:
- How users interact with AI predictions or recommendations
- Where confusion, skepticism, or friction occurs
- Whether the AI output leads to better decisions or actions
This qualitative feedback is just as important as technical performance at the MVP stage.
7. Prepare for Next Steps
Once the AI MVP demonstrates viability:
- Plan data expansion and retraining pipelines
- Architect a scalable version of the solution
- Formalize compliance, privacy, and security reviews
- Build internal or external business cases for full product development
An AI MVP is a starting point—not the finish line.
Common Mistakes to Avoid When Building an AI MVP
- Overengineering – Building unnecessary features or highly scalable infrastructure too early
- Ignoring Data Quality – Assuming a model will work without proper data validation
- Skipping User Testing – Only testing technical performance without involving real users
- Undefined Success Metrics – Launching without clear ways to measure MVP success
- Rushing Deployment – Deploying models before they meet minimum reliability standards
Avoid these mistakes, and your AI MVP will be a true asset—not just another prototype.
Final Thoughts: Build Smart, Build Fast
An AI MVP is the smartest way to de-risk your AI investments. It gives you the proof, feedback, and technical validation needed to scale confidently.
Whether you’re a startup founder, a product leader, or an enterprise executive, investing in a strategic AI MVP can save you months of effort—and millions of dollars—in the long run.
At GlobalNodes, we specialize in building AI MVPs that are lean, practical, and business-driven. If you’re ready to turn your AI idea into a working prototype in just 8 weeks, we’re here to help.